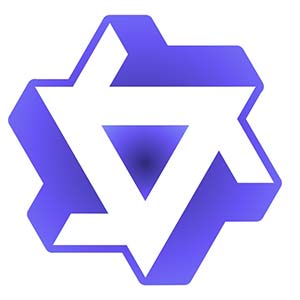
Qwen LLM: Versions, Prompt Templates & Hardware Requirements
Explore all versions of the model, their file formats like GGML, GPTQ, and HF, and understand the hardware requirements for local inference.
The Qwen is a base large language model developed by QwenLM. The series comprises both base language models (Qwen-1.8B, Qwen-7B, Qwen-14B, Qwen-72B) and chat models (Qwen-1.8B-Chat, Qwen-7B-Chat, Qwen-14B-Chat, Qwen-72B-Chat). These models have been pretrained on a vast corpus of multilingual data (public web documents, encyclopedia, books, codes), focusing on English and Chinese, covering a wide array of domains. The model is using a modified version of the Transformer architecture.
Qwen demonstrate competitive performance on benchmark datasets and are capable of tasks such as conversation, content creation, information extraction, summarization, translation, coding, and solving mathematical problems.
Hardware requirements
The performance of an Qwen model depends heavily on the hardware it's running on. For recommendations on the best computer hardware configurations to handle Qwen models smoothly, check out this guide: Best Computer for Running LLaMA and LLama-2 Models.
Below are the Qwen hardware requirements for 4-bit quantization:
For 7B Parameter Models
If the 7B model is what you're after, you gotta think about hardware in two ways. First, for the GPTQ version, you'll want a decent GPU with at least 6GB VRAM. The GTX 1660 or 2060, AMD 5700 XT, or RTX 3050 or 3060 would all work nicely. But for the GGML / GGUF format, it's more about having enough RAM. You'll need around 4 gigs free to run that one smoothly.
Format | RAM Requirements | VRAM Requirements |
---|---|---|
GPTQ (GPU inference) | 6GB (Swap to Load*) | 6GB |
GGML / GGUF (CPU inference) | 4GB | 300MB |
Combination of GPTQ and GGML / GGUF (offloading) | 2GB | 2GB |
*RAM needed to load the model initially. Not required for inference. If your system doesn't have quite enough RAM to fully load the model at startup, you can create a swap file to help with the loading.
Memory speed
When running Qwen AI models, you gotta pay attention to how RAM bandwidth and mdodel size impact inference speed. These large language models need to load completely into RAM or VRAM each time they generate a new token (piece of text). For example, a 4-bit 7B billion parameter Qwen model takes up around 4.0GB of RAM.
Suppose your have Ryzen 5 5600X processor and DDR4-3200 RAM with theoretical max bandwidth of 50 GBps. In this scenario, you can expect to generate approximately 9 tokens per second. Typically, this performance is about 70% of your theoretical maximum speed due to several limiting factors such as inference sofware, latency, system overhead, and workload characteristics, which prevent reaching the peak speed. To achieve a higher inference speed, say 16 tokens per second, you would need more bandwidth. For example, a system with DDR5-5600 offering around 90 GBps could be enough.
For comparison, high-end GPUs like the Nvidia RTX 3090 boast nearly 930 GBps of bandwidth for their VRAM. The DDR5-6400 RAM can provide up to 100 GB/s. Therefore, understanding and optimizing bandwidth is crucial for running models like Qwen efficiently
Recommendations:
- For Best Performance: Opt for a machine with a high-end GPU (like NVIDIA's latest RTX 3090 or RTX 4090) or dual GPU setup to accommodate the largest models (65B and 70B). A system with adequate RAM (minimum 16 GB, but 64 GB best) would be optimal.
- For Budget Constraints: If you're limited by budget, focus on Qwen GGML/GGUF models that fit within the sytem RAM. Remember, while you can offload some weights to the system RAM, it will come at a performance cost.
Remember, these are recommendations, and the actual performance will depend on several factors, including the specific task, model implementation, and other system processes.
CPU requirements
For best performance, a modern multi-core CPU is recommended. An Intel Core i7 from 8th gen onward or AMD Ryzen 5 from 3rd gen onward will work well. CPU with 6-core or 8-core is ideal. Higher clock speeds also improve prompt processing, so aim for 3.6GHz or more.
Having CPU instruction sets like AVX, AVX2, AVX-512 can further improve performance if available. The key is to have a reasonably modern consumer-level CPU with decent core count and clocks, along with baseline vector processing (required for CPU inference with llama.cpp) through AVX2. With those specs, the CPU should handle Qwen model size.